by dorota | Aug 9, 2022 | blog
Marketing uses a variety of strategies which purpose is to help companies attract as many customers as possible. The role of most of these strategies is also connected with building strong relationships with customers. Today, we’re going to show you one of the most effective customer satisfaction strategies – marketing personalization. What is personalization? And how can you use it in your business? Let’s find out.
The term personalization itself is used widely, even in everyday situations; take, for example, your best friend’s birthday. While preparing a gift, you would like to make it special – and special means aligned with your friend’s interests, passions, or dreams. In other words, you want to give them a personalized gift. Even though the meaning of personalization may seem obvious, there are a few things worth clearing out. In this article, we would like to uncover this concept by discussing its definition, benefits, and examples.
What is personalization?
Marketing personalization is a process of gathering customer data (especially about customer behavior) and, based on that input, tailoring the information which the company wants to convey to its customers. Personalized marketing is based on understanding and fulfilling customer preferences, needs, and expectations (that’s how customer segments are created). This way, it is possible to create a message which will be a perfect fit for each and every person involved in the company’s services.
Marketing personalization is a highly important and popular technique that helps you to attract and keep as many customers as possible. We all want and desire to be noticed and understood, and this is exactly what personalized content shows us – attention to individuals visiting your web page.
There are two most common types of personalization used in marketing: one-to-one personalization and segmentation.
The first one is also called “individualization”, and it is the type that is usually associated with the personalization term. In short, it takes into account individual customers, their needs, and preferences and leverages customer data to craft a personalized message.
Segmentation, on the other hand, takes into account groups of customers identified from a bigger audience. The message is not addressed to individuals, then; rather, there is a personalized message for a specific group (customer segment). The best example here would be regular customers who get special information tailored only to their experience. This method, however, can be successful only if the targeted segments are big enough to produce satisfying outcomes.
Why is personalization important?
Personalized experiences seem to be important both for customers and marketers. There are many reasons for which companies introduce personalized messages; the most crucial, however, is the fact that customers nowadays expect to be recognized and gratified for being a loyal part of a brand. Many surveys have been conducted, and all of them present the same results: The more the company is able to notice and identify a particular customer, the higher the chance that the given customer will continue using their services. Of course, it is not the only reason why personalization has become such a successful marketing technique. What else does it involve?
It helps your business thrive. When the customer notices well-tailored messages and feedback, they are more likely to come back and use the company’s services again. That is why it is important to pay attention to the experience your customers encounter while cooperating with your company. One of the biggest names now, such as Netflix or Spotify, owes their success mostly to their personalized playlists. To be fair, the users of these platforms demand personalized content on the basis of which they can find another, well-tailored one.
Personalization vs. customization
Both personalization and customization involve modifications within a customer’s experience. There is, however, a very important difference between these two approaches.
As stated before, personalization includes gathering information about a specific customer. And on this basis, the company proposes specially tailored messages. This way, the customer does not have a direct influence on the content and suggestions that they receive.
With customization, on the other hand, it is the customer who is in charge of modifying the content according to their needs and preferences. While shopping online, for instance, customers receive both personalized and customized content – ads and filtered products, respectively.
Personalization examples
There are many ways in which companies can convey their personalized messages. Mostly, they involve machine learning algorithms that gather information about customer experiences, preferences, likes, but also about information related to the location or online activity. All of this can help companies create as much personalized content as possible. Here are a few most popular personalization methods:
- E-mail personalization
- Smart product recommendations
- Advertisements based on customer data
Each of them can have its own benefits; that is why your company needs to recognize your customers’ needs in order to prepare the best, most satisfying, and well-tailored content.
by dorota | Aug 9, 2022 | blog
Customer engagement is one of the buzzwords of the modern business world. And for a reason – having customer engagement enables you to grow your business, sell more, and even introduce new products and services. In this article, we’re going to show you four bulletproof customer engagement strategies that you can use in 2022. Let’s get right to it.
Probably every business owner wants to have engaged loyal customers that buy and return frequently. However, customer retention is one of the biggest challenges of today’s business world. How can you deal with it? With a solid customer engagement strategy, of course. Your every action has to be purposeful and well-thought-out.
What is a customer engagement strategy?
In short, a customer engagement strategy is a plan that aims at involving your customers into communication with your brand. To do that, you need to tick several boxes. For starters, you need to provide high-quality products. Secondly, your offer has to be relevant to modern consumers. And thirdly, you need a positive image of your brand, something that will allow you to stand out from the crowd. Today, customers value brands that are foremost authentic.
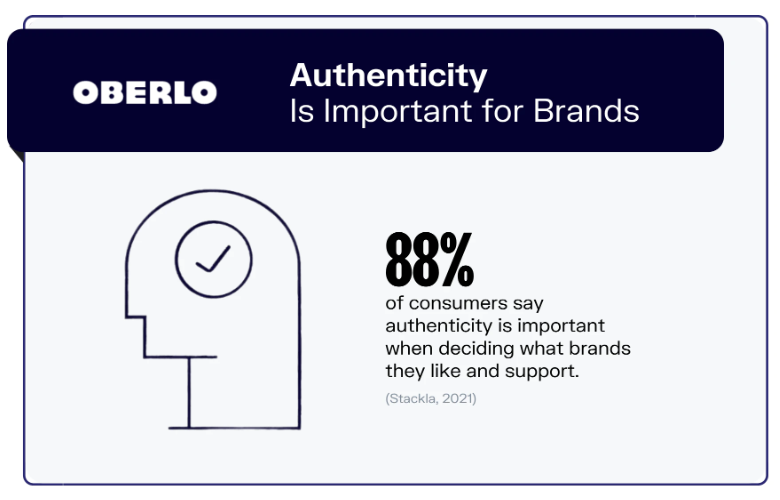
image source: https://www.oberlo.com/blog/branding-statistics
When you get there, you can think about a comprehensive customer engagement strategy. Without all the elements we mentioned earlier, you won’t be able to retain customers for long.
Why is customer engagement important?
Primarily because it enables your business to grow. Brands with engaged customers are more stable, develop even in uncertain market conditions, and simply sell more than other companies that neglect the issue of customer engagement.
You can maintain strong relations with customers with a decent customer engagement plan. And when they trust you and want to communicate with you, they will follow you almost everywhere.

The best engagement strategies for growing businesses
There are many ways to show customers that you value them and to engage them in a conversation with your brand. The vast majority of customer engagement strategies begin with customer data. Yes, you need to know your customers and their needs and answer them as well as possible. Below, you will discover four effective customer engagement strategies. What do you need to know about them?
Use analytics data
We frequently say that nowadays, marketing is data-driven. This means that we don’t guess what to do to get more customers. We use available information and, based on that input, we know what works and what doesn’t. It goes further as customers simply expect you to know them. In a Gladly’s report from 2020, customers indicated three of the most important things brands should know about them:

To get that information, you need analytics software that will track your customers’ activity and gather all the necessary information about customers/users. That’s your starting point in every customer engagement strategy.
Know customer journey
You need to know your customers, but also the path they have to walk in order to buy something from you. It is helpful to map such a journey (or journeys) and indicate the most important stages of it, from interest to post-purchase service.
Today, more and more often, these customer journeys are divided into four stages, especially in the e-commerce world. These stages make up a framework introduced by Avinash Kaushik, one of the Google experts. It’s called See-Think-Do-Care and it shows how the customer journey works. You can read more about it here.
Loyalty programs for customers
Many brands, including e-commerce ones, decide to start a loyalty program. Usually, it’s a good idea. With such a program, you can gather even more information about your customers, but above all, make them feel special. Loyalty programs or VIP clubs create a sense of being a part of something exclusive and limited. Thanks to these clubs, brands can introduce new products, offer special discounts, and share additional benefits.
Personalization
Personalization is a regular guest on our blog. That’s because this strategy is immensely effective. You can personalize almost every element of your offer:
- Product recommendations
- Communication with customers and marketing campaigns
- Products and services themselves
- Content you produce
- Website and mobile apps
The goal is to make people feel taken care of. No one wants to feel like a statistical number, right? When you introduce personalization to your offer, you show customers that you value them and want to help them solve their problems or achieve their goals. Surely, people feel much better entering a website that greets them and shares some essential news, like, for example:
Hey Peter, it’s great to see you again! Check out our new arrivals.
This strategy is simple but extremely effective. That’s why it’s more and more common and not just in the e-commerce sector.
We showed you four useful tactics that can be a part of your strong customer engagement strategy. Implement these elements into your offer, and you’ll see the results!
by dorota | Aug 8, 2022 | blog
Running an e-commerce store is much work, but you already know it. You experience it every day, from marketing and communication to fulfillment and shipping, not to mention all these repetitive tasks, returns, and customer management. And everything else in between. Unfortunately, sometimes it may seem like you are doing many little things to keep the business going, so you don’t have time to focus on the overall picture to take your business to the next level.
That is where e-commerce automation comes in. Imagine what would your day look like if something just happened without all the tedious work? That’s what e-commerce automation tools offer.
What is e-commerce automation?
E-commerce automation uses tools, applications, software, and systems to automate common manual workflows and tasks. Whether it’s the internal triggering of notifications and rules for important e-commerce metrics, launching personalized emails, or being able to manage and optimize various campaigns in real-time, there’s no doubt machine learning and automation are necessary for a growing online store.
How does e-commerce automation work?
In e-commerce, automation consists of a series of configured actions automatically. This e-commerce optimization technique has three components:
- The trigger event or indication that initiates automation
- A condition that determines the content and course of automation
- The result that corresponds to the performance of the activity without human intervention
There are many processes from the moment the customer places an order until the order is delivered to them. The order is first taken over by the e-seller and processed; then, the purchased product is picked up, packed, and shipped. Many of the assignments that take place during this time can be automated.
Why automating business is important
You can increase sales and encourage brand loyalty among potential and new customers with automation software and tools. Automating business is flexible and can quickly adapt to all business needs. Whether you want to launch email campaigns, social media campaigns, or set up a complex sequence of marketing efforts, the automation tools work hand in hand with your needs to help you achieve what you want.
More and more people shop online, which gives e-commerce an advantage over traditional stores. However, consumers constantly are raising their expectations for faster and more convenient deliveries. As a result, business owners must find ways to act quicker and remain flexible to stay competitive.
The benefits of using e-commerce automation
Thanks to e-commerce marketing automation, you can coordinate all your marketing channels so that they work closely together and help you achieve your business goals. However, this is not the only advantage of automation. Let’s look at some examples of the benefits you can gain by implementing e-commerce automation in 2022.
IMPROVED EFFICIENCY
Every task performed by your e-commerce team is essential. However, some, such as archiving and data entry, are often repetitive, time-consuming, and human error-prone. And despite the implementation of rigorous workflows, nothing allows you to complete these operations very quickly or completely prevent blockages or slowdowns. Automation avoids these obstacles. With it, almost all manual tasks are digitized. As a result, you benefit from guaranteed efficiency. Everyone saves time that can be spent on other jobs and strategic assignments with more added value.
MORE ACCURATE DATA
In e-commerce, rigorous data management is essential. Indeed, a massive amount of data is constantly generated and is continually evolving. If this data is managed manually, there will always be a risk of human error in handling it. That is why automation is essential. Thanks to automatic data management, the risk of potential human errors is significantly reduced and all the resulting consequences.
IMPROVING CUSTOMER LOYALTY AND SATISFACTION
More and more customers are looking for memorable and hassle-free purchases that save time and money. However, they also want to track their orders, receive up-to-date information, including inventory, and manage shipping and returns. You can enable them to access this up-to-date information in real-time, independently, and within seconds by automating business processes. It will satisfy them and make their experience positive and enjoyable. This way, companies build customer loyalty that’s solid and long-lasting.
There are many more benefits of e-commerce automation, but it is essential to choose an efficient and flexible solution to enable the implementation of all necessary automation processes. That is one of the keys to the success of your e-commerce project in 2022! Of course, the engine itself will not do all the necessary work. Effective implementation of automation requires the cooperation of internal teams with an integrator who will ensure that the new system is implemented quickly and without any problems and that it suits the needs of your business.
We know it, and that’s why at RecoAI, we provide the integration service of our recommendation systems. Find out more about our platform or contact us, and we will happily answer all your questions.
by dorota | Aug 8, 2022 | blog
Today, video data is virtually everywhere – from YouTube videos to quality control and surveillance. Intelligent algorithms help us create, analyze, and process video data just like it happens with any other type of digital information. In this article, we will show you how two AI-related technologies – deep learning and machine learning are used when it comes to video data.
Firstly, we need to explain what we mean by video data. To explain that, let’s start with something simpler – a single image. It’s a piece of data that comprises specific dimensions (height and width) and three-color layers called RGB. Put all that together, and you end up with a single image file. Now imagine that you have thousands of such files, and they are temporally ordered – the next appears right after the previous one. Such files are referred to as frames. A collection of such frames constitutes video data. Every YouTube video, every surveillance footage, and every commercial works the exact same way. Moreover, video data is directly linked to another AI technology called computer vision. So every autonomous vehicle also generates tons of video data, continually flowing as it drives around.
The use of video data in relation to AI
Now, how can we use AI-related technologies to manage video files? There are a few options:
- Cognitive systems: They are used with autonomous devices and vehicles. These systems enable robots and vehicles to detect objects in front of them and react accordingly (e.g., to bypass an obstacle).
- Metadata recognition: It can be used to ensure content compliance, e.g., to verify that this particular part of the footage was made on the given day, at the given hour.
- Intelligent solutions: Here, we can mention smart quality assurance at factories that need no human supervision to verify the quality of the products that are being manufactured.
- Image analysis: Frequently used in the healthcare sector, where intelligent algorithms can analyze footage made during examination (e.g., ultrasound) or surgical procedure.
With this introduction done, let’s have a look at these two technologies – deep learning and machine learning.
What is machine learning?
Machine learning is one of the essential AI technologies that is all about machines (algorithms) learning themselves. We start with an initial (so-called training) dataset and a machine learning algorithm that’s supposed to do something based on that dataset – analyze, modify, look for specific elements, etc. We train our algorithm using data from that dataset. Based on that training, the algorithm is capable of doing the same task on new datasets.
Suppose you want to train a machine learning algorithm that will be able to detect product defects on the production line. As a start, you need a dataset comprising faulty products (the more, the better). Such a dataset should contain high-quality images indicating specific defects. The algorithm learns based on that and, thanks to that training, can detect defective products on its own – e.g., by detecting deviations from the norm.
How to use machine learning for video
As you already know, it all starts with a dataset comprising relevant elements that we want to train our algorithm with. But that’s not sufficient. Here, we hit another bump in the road called data annotation. Your data needs to be labeled in a way that teaches the machine learning algorithm how to execute a specific task. This means tagging or labeling these datasets with the characteristics you wish your machine learning algorithm to identify. In other words, if you’re working on a defect detection system, you need a dataset that shows faulty products and indicates specific faults. This way, the algorithm will know that you want it to look for similar defects and inform about them.
What is deep learning?
Deep learning is a more advanced form of machine learning. There is one critical difference between them, though. Machine learning algorithms almost always require structured/labeled datasets (for training purposes). On the other hand, deep learning doesn’t need such extensive training. This technology uses artificial neural networks (that imitate the way the human brain works) to make decisions and analyze input data.
How to use deep learning for video
Undoubtedly, deep learning has revolutionized both AI and computer vision and made these technologies scalable. Deep learning facilitates the data extraction process by using huge sets of training data and multiple training cycles to train algorithms to do a specific job. As opposed to the manual extraction of features (in machine learning), deep learning algorithms automate the entire process and automatically extract necessary parts and elements.
Thanks to deep learning, computer vision algorithms can increase the level and complexity of their learning and deliver satisfactory performance and efficiency. Deep learning is used in processing medical videos, manufacturing quality control, military operations, autonomous vehicles, smart security surveillance, and many other applications.
by Redaktor | Apr 12, 2022 | blog
Recommendation system algorithm – understand and fulfill your customers’ needs
The success of every online company lies in understanding users’ needs and answering them. One of the ways to achieve that goal is through recommendation algorithms that learn user preferences and, based on that input, generate recommendations that are accurate and tailored to a given person. But how does the recommendation system work? What are recommendation engine algorithms involved? And finally – what’s the end result that you can expect? Let’s find answers to these vital questions.
The importance of recommendation systems is especially visible in the e-commerce sector. In the last year’s edition of Gladly’s CX report, almost 80% of respondents said they highly value personalized service.
Moreover, 91% of consumers say they are more likely to shop with brands that provide offers and recommendations that are relevant to them1. This means that if you want to win your customers’ engagement, you simply need to provide them with a recommendation system that offers smart product suggestions tailored to each user’s preferences. Of course, such a system requires a lot of information and support from several AI-related technologies. Moreover, there are at least three models to choose from, so the question of finding and implementing the optimal recommender system requires further explanation. And that’s what we’re going to do right now.
How does the recommendation algorithm work?
Generally speaking, these algorithms analyze customer data (e.g., previous purchases, visited subpages, products added to the cart, search logs etc.) and, based on that, show personalized recommendations comprising products and services that have a good chance of interesting a given person.
Typically, the process consists of five stages:
- Data collection: Recommendation system should gather all the necessary interactions between users/customers and items. Sometimes even not obvious events like navigating search results or interactions with images should be taken into account.
- Data storage: As your online store grows, the amount of available customer data becomes more and more comprehensive. In order for the recommendation system to work, all that data has to be stored for future purposes.
- Data analysis: This is where the magic happens. Intelligent recommendation algorithms analyze all the gathered information so that it can be used to provide customers with accurate product suggestions.
- Data filtering: Candidates for recommendation are generated using various strategies.
- Candidates ranking: In the last stage, machine learning algorithms learn how to rank users
There are three technologies that are indispensable in every recommender system:
- Machine learning: It’s an AI-related technology that uses diverse models and techniques to assess whether the user is going to like the item that’s being suggested. The more data ML can proceed with, the more accurate it becomes.
- Natural language processing: NLP is all about enabling the recommender system to “understand” what the user was looking for or what they typed in the search box.
- Deep learning: DL is a more advanced form of ML that’s capable of analyzing and processing non-linear data (a form of data that doesn’t have a traditional structure like a table or a matrix but something more complex like a list of events or even images or text). Not every recommender system uses this technology, but the ones that do are usually more effective and accurate in their predictions.
Which algorithm is used in recommendation systems?
The first thing you need to know is that recommendation algorithms need to be implemented according to the needs of your business. Recommendation systems are not “one-fits-all” solutions. There are many possible combinations and types of systems that can be implemented. In most cases, we can talk about three major recommendation system algorithms.
CONTENT-BASED RECOMMENDATION SYSTEM
It’s the most standard form of a recommendation system algorithm. In content-based filtering, the algorithm uses product features to recommend other items similar to what the user likes, based on their previous purchases. There are some limitations to this system, though. If the given user buys only sporting equipment, the content-based recommendation system will be able to offer other sporting equipment only.
COLLABORATIVE FILTERING RECOMMENDATION SYSTEM
This model is more advanced. Shortly put, collaborative filtering uses similarities between both products and users (simultaneously) to provide recommendations. In other words, the algorithm analyzes the interests and behaviors of users with a similar profile and the items bought in the past by the given person. Then, they suggest new items based on that thorough analysis.
That’s how Netflix works. Its collaborative filtering algorithms use a user movie rating matrix to suggest new movies and TV shows to other subscribers. Let’s see how that works based on a fictional example. There are two users – you and Mark. Mark watched the Netflix show Space Force and liked it very much (he watched the whole season within two days and gave it a 5-star rating). In the past, you and Mark watched and liked a lot of the same movies and shows. Netflix recommendation algorithms discover that Mark liked Space Force, and they suggest it to watch for you, too.
HYBRID RECOMMENDATION SYSTEM
Hybrid recommendation system like described is only one type of mix between various techniques.
The third recommendation system model is simply a combination of the content and collaborative filtering method. In most cases, it’s based on adding the capabilities of the collaborative filtering method to a content-based approach (and vice versa). It can also exploit content and collaborative-based methods to generate separate predictions or combine them into one comprehensive product suggestion.
How to implement a recommendation system algorithm
Here, you have several options. There are some ready-made recommendation platforms that exploit various algorithms to provide your users with product suggestions. However, such algorithms are not always 100% effective as they are ready-made solutions, and they usually take a lot of time and effort to implement. If you’re after something adjusted for your business, its customers and their needs, you need an experienced partner.
We are a company behind an advanced context recommendation system called RecoAI. Our platform is used and appreciated by tons of clients, and it won the 2019 RecSys competition. It still is in 1st place at this prestigious competition! That’s because our system is effective, versatile, and super-fast. We rely heavily on historical data, but when it comes to new users with no history, we use real-time analysis of the user’s behavior so that our algorithms can provide instant suggestions. Recommendations are adjusted within five milliseconds to ensure top-notch accuracy thanks to advanced machine learning algorithms. Furthermore, with RecoAI, recommendations can be different (articles can be recommended to products, products to videos, influencer posts to products, etc.). And don’t worry; we didn’t forget about privacy issues. At no point any sensitive personal data is processed.
To sum up, with our help, you will get a fully functional product recommendation system and comprehensive implementation support. As a result, the implementation process takes just about two weeks. Drop us a line for details.
- https://www.forbes.com/sites/blakemorgan/2020/02/18/50-stats-showing-the-power-of-personalization/?sh=3994f5a52a94
by Redaktor | Apr 9, 2022 | blog
Recommendation system machine learning – improve the accuracy of your product suggestions
Today, recommendation systems are in a multitude of places and online services. They are common in video streaming platforms, online stores, marketplaces, and even social media platforms. That’s because they make users’ lives easier and help save a lot of time. The accuracy of recommender systems lies in one primary technology – machine learning. In this article, we will show you how recommendation systems work and what role machine learning plays here.
Before we explain how modern recommender systems use machine learning algorithms, let’s talk about the idea for a few moments. You run an online business, perhaps an online store. Why do you need a recommendation system? Does such a solution have any particular impact on your business? The answer is yes. And here’s why.
Why do you need a recommendation system?
In early 2020, Microsoft published an article on 3 prescriptions for subscription success. Here are some of the most interesting findings concerning regular customers that we want to share with you. As it happens, loyal customers are:
- 5x as likely to repurchase
- 5x as likely to forgive
- 4x as likely to refer
- 7x as likely to try a new offering
That really says a lot, doesn’t it? And the whole point of using a recommendation system is to get more loyal customers! Because once you win user engagement, a relationship begins to form. Recommender systems aid you in nurturing these relationships. And bear in mind, such customers are 7x as likely to try your new offer, i.e., your product recommendation! This means that when you have an effective recommendation system in place, you make more money and close more deals every month.
What can a recommendation system recommend?
Although recommendation systems are rather a B2C thing, it doesn’t mean that B2B companies can’t utilize this solution. It really all depends on the niche that you operate in and… a bit of creativity.
Usually, recommendation systems are used to suggest:
- Additional services (e.g., express delivery or gift wrapping)
- Complementary products (that’s what cross-selling is all about)
- New products that a given user should be interested in
- New videos, TV shows, movies to watch (as well as books to read)
- Tools or applications worth trying
- Content shared by a specific user’s friends or profiles that they liked in the past
Recommendation systems can also work across multiple types of items. For example, it’s possible to recommend e-commerce products in editorials or vice versa.
As you can see, they are quite versatile. And thanks to several AI-related technologies (primarily machine learning), they are effective and accurate, too! Let’s talk about that for a few moments.
How do you create a recommendation system in machine learning?
Creating a recommendation system using machine learning on your own is only for large companies that have the necessary knowledge and resources to deal with such a complex assignment. You have to have at least one person experienced in machine learning, for starters. Then, it would help if you had someone to gather and prepare data for training and analysis purposes. And lastly, you need a team that will put all that together, integrate with your online business, and continually improve the end solution as the amount of available data increases. All in all – quite a lot of work.
Of course, there are available solutions that help you build an ML-based recommendation system from scratch, but it still requires a lot of expertise. These are so-called batch recommendations. One of such solutions is TensorFlow.
However, if you don’t have an in-house machine learning team, you can opt for the second option and work with an external AI company such as RecoAI. We work with online businesses and help them design and implement fully-fledged recommendation systems that are compliant with their development strategies and user needs.
Moreover, our clients frequently need to test what recommendation strategy is optimal (after all, there are at least three models to choose from, we’ll get to that in a moment). A strategy pool tries to select the best strategy for any given scenario. A good example can be a product page. If you have a customer with a non-empty shopping cart – often it is much better to recommend items for the shopping cart instead of showing similar products.
In such a situation, we offer Strategy Builder that works in a drag-and-drop setup. With it, our clients can simulate diverse strategies without the need to put them online. They can choose the option that suits their needs, and once the decision is made, we deploy the final version of your brand new recommendation system.
3 popular recommendation systems with machine learning
Before we show you these three models, let’s talk for a few moments about why machine learning is so important in recommendation systems? You see, decent recommender systems generate recommendations based on what they know about each user. So it all starts with thorough data analysis. Let’s use a simple example. We have an ordinary e-commerce customer; let’s call him Frank. Frank has already made several purchases in your store with electronics. He bought a gaming laptop, a racing wheel, and two car racing games. Based on that input, you can think of other products that he might be interested in, right? For starters, you can offer him every new car racing game in your store. You can also try to suggest to him other genres of games, e.g. shooting games.
You as a human know this because you use a super-advanced brain that processes a lot of information. AI algorithms need to learn that from scratch, and that’s what machine learning is for. With this technology, your recommendation system can understand what each customer is interested in and what products to suggest. The more information they gather, the better they become. As a result, your product suggestions will be more tailored to each user’s needs and interests over time.
There are three basic forms of machine learning recommendation systems. Let’s analyze them more thoroughly.
CONTENT-BASED FILTERING
It’s the most straightforward form using machine learning algorithms. Content-based filtering uses product features only to recommend items similar to what the user liked or bought in the past. So our hypothetical customer Frank will only get suggestions featuring car games and maybe other accessories for car games but no other products related to computer games.
COLLABORATIVE FILTERING
Collaborative filtering is more comprehensive, and it uses similarities between both products and other users to provide product suggestions. So in our Frank example, the algorithm will analyze what he bought in the past, but also what other computer game fans like him were interested in. As a result, the scope of recommended products is much wider.
KNOWLEDGE-BASED RECOMMENDER SYSTEMS
Probably it’s the most specific type of recommender system that’s based on explicit knowledge related to recommendation criteria. In other words, such a system decides which product should be suggested in which context. So, if your customer bought a racing wheel, the knowledge-based recommender system will instantly recommend purchasing a car racing game, no matter what that person had purchased earlier.
Another type of knowledge-based recommendation can be some kind of an online guide where the client fills out their criteria and the system presents users with the best options to consider (so-called explicit recommendation system).
On the other hand, the first two systems are more implicit. They do not ask the customer about their preferences but assume what they want based on the previous interactions.
The second option could be better for a limited item catalog, but for a catalog with hundreds or thousands of items, building questionnaires for each product category can be very costly. The RecoAI recommendation system is implicit in this sense because it recommends products based on customer interactions (and they can be very diversified; there are 16 interaction types in our database).
If you want to know more about recommendation systems – feel free to reach out. We will gladly help you get to different users in the most effective way!